What is AI in market research?
AI in market research refers to the use of artificial intelligence tools to gather, process, and analyze complex market, user, and buyer data. These technologies help researchers identify key themes, detect emerging trends, and uncover deeper insights that inform strategic decisions.
When integrated thoughtfully, AI enhances every stage of the research process—from drafting interview questions to analyzing responses and presenting findings. By automating time-consuming tasks, AI allows researchers to focus on what they do best: applying critical thinking, interpreting nuance, and connecting insights to real business challenges.
The true value of AI emerges when paired with human expertise. This combination of automation and strategic judgment enables organizations to move faster, dig deeper, and make more confident, insight-driven decisions.
How do human researchers and AI work together effectively?
AI has become a powerful enabler in market research, streamlining data processing, automating transcription, and helping identify patterns at scale. But while AI delivers speed and structure, human expertise brings context, adaptability, and strategic thinking to the table.
At Cascade Insights, our concept of Fluid Intelligence™ captures the vital human strengths that complement and elevate AI-driven market research. This adaptive framework recognizes that while AI excels at analyzing vast datasets and uncovering patterns, it lacks the nuanced understanding, strategic foresight, and contextual judgment that human researchers uniquely provide. Fluid Intelligence™ emphasizes the human capacity for intuition, adaptability, and empathy—qualities essential for interpreting findings, spotting contradictions, and translating insights into meaningful strategic direction. Ultimately, while AI sharpens our analytical edge, it is human insight that ensures research remains relevant, resonant, and actionable.
In short, it’s not a matter of choosing between AI and human analysis; both are essential, and they work best in combination. Here’s how we’re able to leverage AI for deeper insights:
AI Alone Misses What Truly Matters – We Don’t
Great research isn’t just about collecting words or soundbites. It’s about listening with empathy, paying close attention to your words and tone, nonverbal cues, and what is unsaid as much as clearly stated. Throughout the entirety of the project, our consultants bring:
- Emotional intelligence & strategic thinking – AI detects patterns, but human researchers interpret intent, emotion, and business implications. We refine insights by probing emerging trends, a nuance that would require specialized AI modeling.
- Adaptive methodology – AI tends to follow predetermined paths; we adjust the line of questioning based on participant screener details, live responses, digging deeper where necessary. In short, we continuously refine our approach based on emerging insights, pivoting research direction when unexpected opportunities arise.
- Tech sector expertise depth & context awareness – AI summarizes; we challenge assumptions, validate insights, and connect them to real-world trends.
- Bias detection and correction – Our researchers detect uncertainty, subtext, and hidden biases all of which can lead a research project astray.
How We Use AI Here: AI helps us organize and analyze conversation data more quickly, but human expertise ensures we interpret insights accurately, avoid misleading conclusions, and uncover the deeper meaning behind responses.
Go Beyond a Response: Establish a Connection
Collecting responses isn’t enough; you also need the ability to probe, challenge, or interpret responses within a broader strategic framework.
- AI takes a response at face value – humans recognize subtext. A skilled interviewer can tell when a respondent is holding back, contradicting themselves, or revealing something indirectly.
- AI lacks skepticism – humans challenge assumptions. While AI can be programmed to question, it inherently treats all statements equally. In contrast, human researchers continuously question, validate, and refine findings.
- AI organizes information – humans extract meaning. AI can cluster responses, but only humans can determine which insights are truly valuable.
How We Use AI Here: AI assists with note-taking, transcription, and sentiment analysis, allowing our researchers to focus on engagement and active listening.
Transforming Data into Strategic Action
AI processes vast amounts of data at lightning speed, but data without context is just noise. Our human expertise ensures:
- We distinguish correlation from causation. AI can explain the relation between X and Y; we help you determine why those distinctions matter in your unique context.
- We prioritize insights for business impact. AI reports all trends equally, but not all insights deserve equal attention.
- Strategic foresight. AI analyzes information; we position findings within the competitive landscape of your specific market..
How We Use AI Here: AI helps with trend identification, data visualization, and summarization, but final analysis, prioritization, and strategic recommendations are always human-led.
Secure and Transparent Research
AI can accelerate research, but it must be carefully managed and interpreted to ensure safety and effectiveness. That’s why we blend AI’s power with human expertise to deliver research that is both fast and reliable.
Unlike AI-driven black-box models, our research is fully transparent and verifiable.
- Every insight is traceable to a real source. No bots and No Fraud. No hallucinations, no fabricated conclusions.
- Active bias monitoring. We critically examine both human and AI-generated biases throughout the research process.
- We ensure compliance with research ethics and privacy standards. AI alone cannot ensure full data protection and consent—our human oversight does.
How We Use AI Here: AI accelerates data processing, report drafting, and thematic analysis, but every AI-generated insight is reviewed, refined, and validated by experienced researchers.
Innovate with a Human
AI is exceptional at analyzing past data, but it struggles with true innovation.
- AI interpolates; humans extrapolate. AI can only recombine what it has seen; our researchers identify novel opportunities others miss.
- AI predicts trends; humans create them. The most impactful innovations come from human intuition, creative leaps, and lived experiences.
- A willingness to challenge. AI builds on existing patterns; we question fundamental assumptions to uncover breakthrough insights.
- We challenge conventional wisdom. AI reinforces what’s already known, but true breakthroughs require questioning assumptions.
How We Use AI Here: AI helps identify past trends and correlations, while our human experts explore new possibilities and future market shifts.
Beyond Synthetic: Why Real Human Insights Matter
AI-generated personas and synthetic respondents are increasingly used in research, but relying solely on them instead of real respondents results in insights that lack authenticity, real-world experience, and credibility.
Real respondents provide firsthand insights, uncovering emerging trends and offering nuanced perspectives that AI simply can’t replicate. We use a rigorous, multi-step vetting process to recruit the right real participants, ensuring that:
- Every respondent is manually verified – Every participant is carefully vetted by our research team, not by algorithms.
- We engage experts with firsthand experience – AI can’t replace lived industry knowledge.
- Our sample is strategically balanced – Our researchers ensure diverse perspectives while maintaining methodological rigor.
How We Use AI Here: AI helps us streamline initial screening and organize large respondent lists, but final verification, contextual fit, and recruitment strategy are always human-driven.
AI + Human Expertise = The Best Research
When the stakes are high, you don’t want research that’s simply “good enough.” You need insights that are:
- Fast & data-driven (AI-powered efficiency)
- Deep & strategic (Human-led analysis & expertise)
- Actionable & reliable (Business-focused recommendations)
How is AI used in market research today?
AI is becoming a core component of modern market research workflows. Rather than replacing human expertise, AI complements it—streamlining tasks, accelerating processes, and uncovering patterns in data. Researchers who integrate AI thoughtfully can focus more on strategic thinking while automating many of the time-consuming tasks that traditionally slow down research projects.
Below is a breakdown of how AI supports the research process from start to finish, across both qualitative and quantitative studies.
1. Initial Research and Preparation
The foundation of effective research begins long before any data is collected. In this phase, researchers define the study’s purpose, determine the target audience, and build tools such as screeners, surveys, or interview guides. AI tools can streamline and strengthen this planning process, allowing researchers to move faster while ensuring quality and relevance.
AI Contributions
- Topic and Question Generation: AI tools like ChatGPT or Claude can help brainstorm and refine question sets—whether for a survey or an in-depth interview—based on project goals and audience.
- Technical and Industry Research: AI can scan recent articles, product documentation, and public datasets to provide background information on complex technologies or niche markets.
- Simulation and Testing: Researchers can use AI personas to simulate interviews or survey completions, revealing potential gaps, confusing phrasing, or unanticipated responses.
- Bias Detection: AI tools can analyze wording and question flow to highlight leading or biased questions, improving the quality of the final research instrument.
Human Contributions
- Objective Setting: Researchers ensure the project is aligned with client needs, business objectives, and strategic decisions the research is intended to support.
- Customization: While AI can provide a starting point, human judgment is essential to tailor questions to specific contexts, client concerns, or industry dynamics.
- Iterative Development: Human researchers continuously refine materials in collaboration with clients, ensuring all tools meet project goals and are methodologically sound.
- Recruitment Strategy: AI may assist with list-building or segmentation, but final decisions about who qualifies to participate are made by researchers based on experience and nuance.
2. Data Collection: IDIs, Focus Groups, and Surveys
Data collection is a dynamic process that often requires adaptation in real-time. Whether collecting qualitative or quantitative data, researchers must ensure that interactions are productive, ethical, and aligned with research objectives. AI supports this stage primarily by improving accuracy, reducing administrative burden, and enhancing real-time insight capture.
AI Contributions
- Transcription and Notetaking: AI can provide accurate, real-time transcription of interviews and focus groups, ensuring that no insights are lost and allowing researchers to stay fully engaged.
- Highlight and Sentiment Detection: Some tools can flag key phrases, emotional cues, or shifts in sentiment—either during or after a session—for further analysis.
- Talk Time Monitoring: AI can help manage conversation balance during focus groups, ensuring equal participation across respondents.
- Survey Optimization: During quantitative data collection, AI can detect potential survey fatigue or inconsistent response patterns and flag them for review.
Human Contributions
- Building Rapport: Human researchers create the trust necessary for honest, open responses—especially in interviews or focus groups.
- Adaptive Questioning: Skilled moderators adjust lines of questioning based on a respondent’s tone, clarity, and cues—something AI still struggles to do effectively.
- Ethical Oversight: Researchers ensure that all interactions comply with privacy, consent, and ethical standards.
Contextual Awareness: Humans are better equipped to detect when an answer deserves deeper probing or when an unexpected insight emerges mid-conversation.
3. Data Analysis
AI is significantly impacting how researchers analyze quantitative and qualitative data. These tools are built to efficiently process large volumes of information, uncover key themes, visualize trends, and identify patterns that would be difficult or time-consuming to detect manually. When used strategically, AI enhances—not replaces—the role of the human researcher. These are some of the most common use cases for AI in both quantitative and qualitative data analysis:
AI in Quantitative Research Analysis
- Survey Design Optimization: AI can refine survey structure by suggesting relevant and unbiased questions tailored to specific research goals and audiences. It can also detect and mitigate potential biases, improving data quality and respondent experience.
- Pattern Recognition and Trend Analysis: AI is highly effective at identifying trends, correlations, and emerging signals within large datasets—surfacing insights that may not be immediately obvious. This includes integrating survey responses with other data sources like CRM data or industry benchmarks.
- Anomaly Detection: AI tools can flag unusual response patterns, such as suspicious timing or inconsistent answers, helping ensure data integrity by identifying potential bot activity, fatigue, or respondent error.
- Data Visualization: Interactive dashboards and AI-generated visuals help researchers and stakeholders quickly understand the story behind the numbers. These visuals support faster interpretation and more impactful presentations.
- Predictive Modeling: AI can analyze historical data to forecast future trends—such as predicting customer satisfaction scores based on past response patterns. These models can support proactive decision-making and strategic planning.
- Analysis of Open-Ended Responses: Using natural language processing (NLP), AI can quickly process and categorize large volumes of text-based survey responses. Tools like codeit can automatically extract sentiment, identify themes, and highlight key quotes—making it easier to turn unstructured feedback into structured insight.
AI in Qualitative Research Analysis
- Transcription and Initial Processing: AI can accurately transcribe audio and video recordings from interviews and focus groups. It can also flag key moments, summarize responses, and highlight sentiments or themes—saving researchers time in the early review process.
- Efficient Data Summarization: AI-generated summaries condense lengthy transcripts into key points, making it easier for researchers to grasp high-level findings without losing important details.
- Theme and Pattern Identification: AI helps detect recurring themes and sentiments across interviews and qualitative datasets, supporting deeper and faster exploration of qualitative inputs.
- Sentiment Analysis: AI tools assess tone and emotion across qualitative responses, helping researchers understand how participants feel about a product, brand, or topic.
- Visual Representation of Qualitative Data: Tools can generate visual aids such as word clouds, heat maps, and theme maps that illustrate the frequency and emotional tone of specific terms or topics—useful for communicating findings to non-research stakeholders.
- Contextual Linkage: AI can cross-reference qualitative data with external reports, academic sources, or prior internal findings—offering additional context that helps validate or challenge emerging insights.
- AI-Powered Questioning: Some tools can suggest follow-up questions or lines of inquiry based on previous responses—supporting deeper exploration or triangulation of findings during ongoing analysis.
The Role of the Researcher in AI-Powered Analysis
While AI accelerates analysis, it’s human expertise that ensures findings are interpreted correctly and turned into meaningful recommendations. Researchers play a critical role in:
- Data Interpretation: Reviewing AI-processed outputs, refining focus areas, and making connections that reflect business realities and context.
- Critical Thinking: Evaluating assumptions, exploring alternative explanations, and assessing the implications of the data.
- Industry Insight: Applying sector-specific knowledge to assess relevance, accuracy, and applicability of findings.
- Hypothesis Formation: Synthesizing information across multiple data points to create well-supported conclusions and compelling strategic narratives.
4. Reporting and Strategy Development
Turning raw data into a clear, compelling story is one of the most critical phases of market research. AI tools can support efficiency and structure, but the interpretation and communication of findings—and the translation into strategic recommendations—relies heavily on human insight.
AI Contributions
- Data Summarization: AI can assist in pulling key quotes, summarizing large volumes of content, and organizing supporting evidence efficiently.
- Slide Deck Generation: Tools can help design initial report drafts, create visual assets like charts or diagrams, and organize information in a digestible format.
- Interactive Reports: Some AI-enabled platforms allow stakeholders to interact with findings, exploring different themes or personas in a dynamic way.
- Creative Support: AI can offer alternative phrasing, headline ideas, or framing suggestions for key messages, helping improve communication impact.
Human Contributions
- Insight Prioritization: Researchers determine which insights matter most for business impact, rather than reporting all findings equally.
- Narrative Construction: The human role includes crafting a coherent story that connects the research findings to client goals and strategic questions.
- Presentation and Facilitation: Researchers play a key role in presenting findings in live sessions, answering stakeholder questions, and guiding the next steps.
- Strategic Recommendations: AI might suggest correlations, but it’s the researcher who evaluates the feasibility, weighs trade-offs, and tailors recommendations to the organization’s context.
5. Socializing the Findings
Research findings only create value if they are shared, discussed, and acted upon. This stage focuses on helping stakeholders internalize insights, explore implications, and use research to guide future decisions. AI can support this by keeping the data accessible and interactive—while humans play a central role in facilitating discussion and action.
AI Contributions
- Synthetic Respondent Simulations: In some cases, AI tools can generate simulated personas based on study data to allow stakeholders to “chat with the findings” and explore themes over time.
- Knowledge Retention: AI can create searchable summaries and conversational interfaces to help stakeholders revisit research without reading a full report.
- Exploration Tools: AI-enabled dashboards or chatbots can offer tailored responses to stakeholder queries, helping extend the research’s shelf life.
Human Contributions
- Facilitating Internal Buy-In: Researchers help teams understand, champion, and communicate the research internally.
- Strategic Follow-Up: After findings are socialized, researchers often help with applying them to new messaging, campaign development, product strategy, or sales enablement.
- Ongoing Support: Human researchers are critical in ensuring that findings remain relevant as new questions arise or business conditions shift.
- Contextual Clarification: When findings are misinterpreted or overextended, researchers can clarify limitations and help stakeholders stay grounded in the data.
What types of AI tools are used in market research?
AI tools in market research span a wide range of functions across the entire research workflow—from early planning and data collection to analysis and presentation. Here is an organized overview of the primary tool types and notable platforms within each category, along with how they support both qualitative and quantitative research efforts.
1. Secondary & Desk Research Assistants
AI tools are rapidly transforming desk research by streamlining how researchers explore competitive landscapes, analyze online chatter, and synthesize multiple sources. These assistants are best viewed as a way to accelerate foundational understanding, though they must be used critically.
- Perplexity Pro: Excels at source-limited, focused secondary research across platforms like Reddit, YouTube, and the broader web. Especially useful for gauging public sentiment and discovering community-level narratives.
- Deep Research Modes – ChatGPT & Gemini: Both offer structured canvases for collecting secondary research and generating reports. Gemini excels in transparency and reasoning clarity, while ChatGPT offers greater extensibility via PDF uploads.
- NotebookLM: An AI tool built around stored documents and notes. Its interactive interface makes it easier to explore relationships among themes and generate summaries. Bonus: it can produce audio overviews for quicker consumption.
- Lindy: Tailored to automating workflow integration, Lindy connects datasets, pulls from shared files, and delivers auto-summaries via defined logic. Ideal for repeated tasks like meeting prep, trend monitoring, or summary generation.
These tools can significantly reduce the time spent on early-stage research, especially when surfacing patterns across unstructured sources. But while they accelerate information gathering and trend discovery, they’re not infallible. Researchers must still vet the outputs carefully—particularly when they’ll inform high-stakes strategic decisions. Transparency, source attribution, and human interpretation remain essential.
And often, the most valuable outcome of this research is a sharper set of questions – questions that feed directly into the next phase: interview and survey preparation.
2. Interview, Focus Group, and Survey Preparation
These tools help researchers generate questions, simulate conversations, and even create AI-based personas to test hypotheses before interacting with real participants. They can significantly improve early-stage preparation — but still require experienced human input to avoid shallow or overly generalized approaches.
- ChatGPT Team Edition: Enables creation of custom GPTs using uploaded transcripts or persona data. Great for simulating buyer conversations, brainstorming questions, and refining interview guides. Offers strong privacy controls and team management tools.
- Claude: Helpful for refining interview guides and simulating question flows. Its flexibility is strong, though bulk transcript analysis requires careful input structuring due to file limitations.
- Gemini: Offers strong contextual reasoning and a clean interface. Ideal for generating question sets, refining hypotheses, and preparing for stakeholder interviews. Gemini also performs well in summarizing recent online discourse and synthesizing it for survey and IDI prep.
- Deep Research Modes – ChatGPT & Gemini: Both platforms offer structured canvases to help researchers generate secondary research reports, identify hypothesis areas, and build stakeholder-facing briefs. Gemini emphasizes transparency and sourcing; ChatGPT supports uploads and offers a slightly more creative output style. Best used to shape initial framing and strategic direction before data collection.
- Perplexity Pro: Effective for surfacing emerging topics and gaps in current knowledge, making it a powerful co-pilot for early-stage prep. Great for understanding public sentiment or evaluating trending pain points before fielding research.
These tools can simulate responses and explore line-of-questioning scenarios, but they cannot replace the subtle insights uncovered through live human interaction. Their best use lies in helping researchers refine hypotheses and structure more effective live interviews.
3. Conversational AI Interviewers
Voice-based and text-based AI tools are evolving to simulate or conduct interviews autonomously but with caution. These platforms show real promise for automating high-volume, low-stakes interviews (like screeners or early-stage research), yet they currently fall short of replacing skilled human moderators. Also in nearly all cases, the interviewer itself is chat-based with a limited number of the tools in this space providing an audio-based interviewer.
- Strella: An audio and chat-based AI interviewer capable of conducting voice interviews. Its strength lies in simulating natural conversations with real-time transcription, offering scalable voice-based qualitative research.
- Listen Labs: A chat-based interviewer designed to help automate qualitative interviews. Ideal for high-volume interviewing where consistency and speed are prioritized.
- Versive: Another chat-based platform that simulates respondent interviews and gathers input in a structured, conversational format. Particularly suited for replacing structured screeners or guiding exploratory discovery at scale.
These tools provide tangible value today for teams experimenting with AI-conducted interviews—especially in situations where speed and scale matter more than nuance. However, oversight and moderation remain essential.
Simultaneously, the market is rapidly evolving towards audio-first AI interviews. For example, the following concept/platform demos of AI audio platforms and APIs suggest a future where audio-first (and potentially video) AI interviews will become commonplace.
- Sesame: Open-source voice model offering memory, tone control, and fluid back-and-forth conversation.
- OpenAI’s Voice Mode: Demonstrates real-time reasoning and emotional tone variation in voice.
- Hume AI: Adds emotional analysis to conversations, interpreting vocal cues and affect.
For now though, AI interviewers are best viewed as a supplement to human moderation, not a replacement. AI interviewers should be paired with strong oversight, clear disclosure, and robust validation practices. As this space matures, researchers must constantly evaluate where automation of this kind helps — and where it risks undermining the authenticity and integrity of qualitative insight.
4. Transcription & Note-Taking Tools
Accurate transcripts form the backbone of quality qualitative research. AI has vastly improved this space, but not all tools are equal—especially when it comes to niche, technical conversations where clarity and accuracy are vital.
- Fathom: Offers one of the most accurate transcription engines for technical interviews. Standout features include live tagging, structured summaries, and templates for different interview types. Especially well-suited for complex B2B use cases.
- CoLoop: Goes beyond transcription by allowing researchers to “chat with” their data. Powerful for extracting quotes, summarizing by theme, and seeing responses in context. Its analysis grid provides a structured overview of participant responses.
- Zoom-native bots (e.g., Otter.ai, Fireflies.ai): Many meeting platforms now offer AI companions that can transcribe, summarize, and tag action items. These bots are easy to deploy and integrate well into day-to-day workflows—but their output often struggles with domain-specific terminology and may require significant editing.
Researchers should still review transcripts carefully for errors—especially in specialized domains—but tools like Fathom and CoLoop substantially reduce the manual burden of analysis prep and enable deeper focus on synthesis.
5. Data Analysis Platforms
AI-driven platforms have revolutionized how qualitative and quantitative data are processed, categorized, and visualized. These tools can uncover key themes quickly, but expert oversight remains critical for interpretation.
- codeit: Designed for survey open-end analysis, codeit offers powerful auto-coding and theme identification. Researchers can tune auto-coding thresholds and review AI-generated codes, ensuring high confidence in the output.
- CoLoop (again): Offers visual exploration of themes and sentiment. Its AI grid helps tie insights directly to transcripts, making pattern discovery and presentation more intuitive.
- Claude: Effective for summarizing large text inputs, comparing transcripts, or identifying patterns across open-ended responses. Claude also excels at developing charts and visualizations of existing datasets.
- Julius.ai: Tailored to data visualization and quantitative analysis, Julius.ai can process structured datasets, create charts, and summarize numerical findings — helpful in translating raw survey data into insights. Best used as a co-pilot, not a final authority on analytics.
These tools are indispensable for scaling analysis across large data sets. But researchers still need to apply critical thinking to distinguish signal from noise, interpret ambiguity, and align findings with the strategic context of the study.
6. Writing & Insight Communication Tools
Communicating research clearly and persuasively is just as important as conducting it. AI writing tools help translate insights into digestible content—but they work best when paired with human nuance and industry fluency.
- Lex: A writing assistant that helps researchers maintain voice while improving structure, clarity, and flow. Especially strong for B2B reports. Includes readability tools and audience-specific checks to guide tone and complexity.
- NotebookLM (again): Excellent for repackaging longform content into insight summaries, Q&A formats, and slide-ready excerpts. Can help draft narrative arcs for reports.
- ChatGPT, Gemini, and Claude (again): All three are highly effective for generating first-draft content, whether it’s research summaries, executive briefs, or internal emails. Their ability to rephrase insights, adjust tone, and provide creative structuring makes them useful writing collaborators. These capabilities are also integrated into many productivity platforms today, such as Copilot in Microsoft Outlook and Gemini in Gmail, offering in-context writing support for research teams.
These tools reduce the friction between insight generation and stakeholder communication. Still, AI alone doesn’t know what will resonate—human input is crucial to connect insights to strategy, audience, and market needs.
7. Visual and Presentation Tools
AI design platforms make it easier to turn findings into engaging, stakeholder-friendly visuals and presentations. These tools are a productivity boost—but also a creative partner when used thoughtfully.
- Ideogram: Ideal for clean, professional image generation. Especially valuable in B2B contexts where fantasy-driven visuals fall short. Strong text integration and excellent free-tier accessibility.
- Midjourney: Powerful for producing rich, stylized visuals across a range of creative themes. While often more artful or abstract in tone, Midjourney can be valuable for conceptual imagery or mood setting when positioned properly within professional research outputs.
- ChatGPT 4o Image Generation: Offers fast, responsive image generation with tighter control over specific image elements. Integrated into ChatGPT, it makes iterative prototyping easier and accelerates visual asset creation. The recent release also handles images + text extremely well.
- Gamma: AI-powered slide and report creator. Removes the design bottleneck by auto-generating layouts and visuals across multiple formats. Web-first, mobile-ready, and integrates several image tools (e.g., Midjourney, ChatGPT 4o Image Generation,, Ideogram).
Together, these tools enable a modern, AI-enhanced research workflow that supports efficiency, depth, and strategic clarity. Importantly, the best outcomes come not from relying on any single tool—but from thoughtfully combining automation with human expertise throughout the research process.
AI and Human Insight: The Future of Market Research
AI in market research is no longer a trend on the horizon; it’s a foundational part of modern research. From accelerating desk research to streamlining analysis and scaling communication, AI is transforming how insights are gathered, interpreted, and shared.
But despite all its power, AI doesn’t replace what matters most: the strategic thinking, contextual understanding, and real-world experience that human researchers bring to the table.
At Cascade Insights, we champion this synergy. By embracing AI as a powerful tool while upholding the irreplaceable value of human judgment, we deliver research that’s both efficient and profoundly insightful. The future of market research is not about replacing humans with AI, but about amplifying human capabilities with its power. It’s a future where we move beyond data analysis to strategic foresight, driving decisions that are not just informed, but truly transformative. Learn more about our approach to AI-enhanced B2B market research.
A Few of our Clients
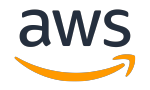
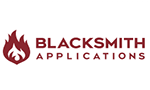
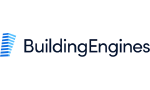
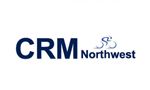
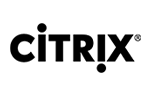
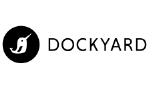
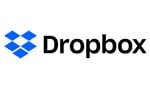
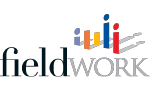
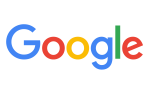
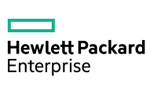
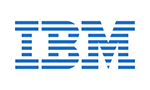
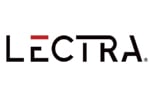
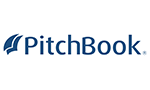
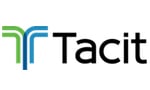
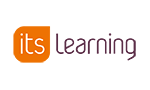
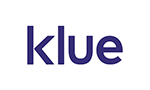
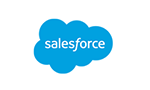
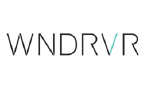